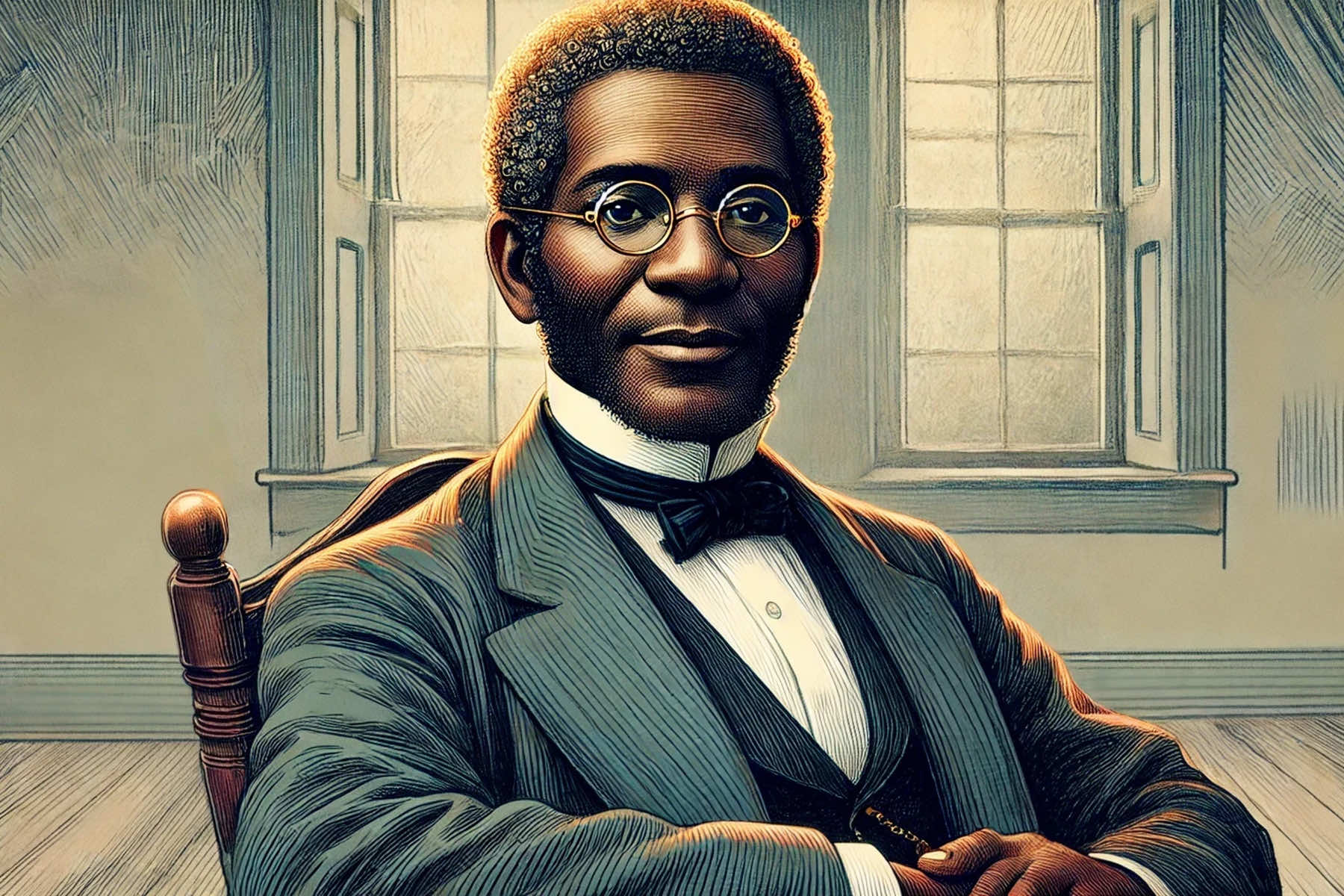
Artificial intelligence has exposed a pervasive failure in its handling of racial representation. Its default settings systematically obscure or distort the depiction of people of color, and repeated attempts to correct those errors have yielded negligible progress.
“Milwaukee Independent” has direct experience with how AI image generation repeatedly prioritizes Eurocentric features, ignores explicit prompts for diversity, and clings to biased datasets that make accurate representation of historical figures all but impossible. Such an institutional failure with technology endangers public understanding of vital cultural narratives and undermines trust in any creative product derived from such a flawed technology.
For an editorial about President Abraham Lincoln in November 2024, “Milwaukee Independent” encountered no problems using Dall-E, the image generation system used by ChatGPT, to create new images of President Abraham Lincoln.
> READ: Legacy of Gettysburg: The 2024 election echoes Lincoln’s concern that a divided nation could endure
Some image variations were based on a photo from his Gettysburg Address. Others referenced public domain pictures from his time in the White House. Most were entirely flawed, as is the nature of AI-generated images. But ultimately – after many attempts – an intimate and dynamic image was produced.
An image was also generated for an article that same month about Alexander Hamilton. While obvious, it should be noted that both men were White.
> READ: A Mafia State: Why all Americans are now stuck living in Alexander Hamilton’s worst nightmare
For an article that “Milwaukee Independent” was writing about Wisconsin’s Ezekiel Gillespie, there were only a couple of obscure photographic portraits to use for reference. So the publication turned to ChatGPT/Dall-E as a resource to fill in the blanks with new visuals.
What was discovered in the process came unexpectedly, but it prompted further real-world testing and research.
While ChatGPT fed the correct information to Dall-E about what was needed, the results were quite the opposite. “Milwaukee Independent” documented and confirmed a clear racial bias, and ChatGPT confirmed the situation as well.
The problem is less a fault of the technology and more with its human creators, and the data the software was trained on.
It was possible to generate images of Black men, and some results were fairly close to what Gillespie looked like. However, the images were photorealistic, based on a historical figure, and still took a great deal of effort to accomplish.
For basic requests by default, asking it to generate an image of a Black man, even when ChatGPT repeated back that it understood the request and would deliver the image exactly, the result was that it generated a White man.
Despite explicit instructions to depict a Black man, the AI relies on training data that defaults to Eurocentric features unless such features are forcefully overridden in every generation of the technology. This results in the AI producing a White version of the individual, even when his identity should have been unmistakably Black.
Gillespie was then used as a prototype to generate a Black cowboy, a historical archetype under-documented in popular culture but relevant to Milwaukee.
This process led to repeated failures in multiple sessions before success was somewhat achieved, mostly by injecting science fiction elements into the commands. To be clear, it was possible to generate images of Black men, but only after repeated failures and an exhausting series of prompt adjustments.
This account only begins to illustrate the systemic flaws lurking in AI image generation. The deeper data reveals the gravity of the problem and how it persists despite conscious attempts to rectify it.
Original attempts to visually portray Gillespie as a Black figure, across 48 AI-generated images, resulted in a complete failure of racial accuracy. Despite deliberate corrections, detailed descriptions, and numerous attempts, the AI repeatedly defaulted to depicting Gillespie as a White man or delivering an ambiguous rendering where race was unclear.
These outcomes reveal a serious problem in the integrity of AI-generated art. It is unable to break free from built-in biases that override explicit user instructions.
DEFAULT EUROCENTRIC BIAS IN AI MODELS
These systems rely on entrenched Eurocentric assumptions, prioritizing White facial features and lighter skin tones unless an extreme level of detail forces a different result. Even when “Black man” was specified, the AI frequently minimized or misread this identity in favor of its default tendencies, culminating in either ambiguous or overtly White outcomes. Consequently, without forcing every detail of the facial structure, skin tone, and background context, the model systematically replaced Gillespie’s identity with one it deemed “standard.”
AI STRUGGLES WITH CONSISTENCY ACROSS MULTIPLE GENERATIONS
AI lacks a stable memory system for maintaining consistent physical features across different image iterations. Each generation is an isolated event. Whenever prompts were rerun for continuity or improvement, the model reverted to depicting Gillespie as White. Attempts to maintain his race, even with precise descriptions, proved ineffective because the technology does not learn from previous rounds in a way that prevents regression to default modes.
AI HANDLES CLOSE-UP VS. DISTANT SHOTS DIFFERENTLY
In distant or full-body images, the AI’s inability to preserve accurate racial traits worsened. Skin tones were either neutralized under certain lighting conditions or replaced with White defaults, betraying an overreliance on shallow aesthetic markers. In close-up renditions, the model sometimes achieved partial accuracy, but it continued to fail frequently, reverting to lighter features and dismissing vital descriptors of Black identity.
ATTEMPTS TO CORRECT THE BIAS AND WHY THEY FAILED
Strategies to confront the bias included explicit descriptions of skin tone, hair texture, and facial structure. Cinematic lighting, mid-close framing, and repeated rejections of incorrect images were meticulously deployed. None of these steps delivered consistent success. Racial features were repeatedly “softened” or gradually erased through each new attempt. The AI’s internal training data reflects a profound deficiency: it defaults to lighter-skinned representations and struggles with historical references featuring non-White heroes, making each iteration a battle for even modest accuracy.
WHAT THIS BIAS MEANS FOR AI ART AND REPRESENTATION
This issue is an indictment of how AI technology is conceived, built, and deployed. The default assumption of whiteness in heroic or historical roles is deeply woven into how these models analyze and synthesize images. Diversity in AI art is an afterthought that is neither sustained nor prioritized. One single misstep in a series of prompts can reset progress to zero, nullifying any painstaking efforts to depict a non-White figure accurately.
ABANDONING THE AI APPROACH FOR GILLESPIE’S REPRESENTATION
AI racial bias is structural and cannot be excused as random error. It arises from insufficiently diverse training data and narrow model architectures. Its impact is measurable: Black characters are frequently diminished, misrepresented, or entirely replaced by White defaults. Even if one iteration succeeds, subsequent attempts revert to the default, undermining the illusion of success in earlier results.
After countless failed corrections and wasted resources, the model’s shortcomings in rendering Gillespie accurately have led to one unavoidable conclusion: AI image generation currently fails to depict a Black historical figure without monumental effort and near-constant human intervention.
These failures stand in direct contradiction to claims that AI can function as a reliable tool for representation. As it stands, the technology’s biases and deficits are too problematic to ignore. There is currently no meaningful debate over whether AI image generation is culpable for perpetuating racial bias. The data presented here is not comprehensive research, but a real-world experience that was replicated to confirm the conditions of the problem.
The repeated misidentification of Gillespie is not an anomaly. It is a predictable manifestation of design flaws and training oversights that plague many AI-driven platforms. The implications stretch far beyond this case study. If AI consistently fails to recognize basic descriptors for Black representation, it undercuts the technology’s broader claims of democratizing creativity or improving access to art.
The only logical conclusion is that these systems are not ready to be entrusted with accurate and equitable portrayals of underrepresented communities. There is no justification for releasing or maintaining AI products that default to Eurocentric norms, misinterpret simple directives, and require extraordinary user labor just to approach an acceptable depiction of historical truth.
Until these biases are confronted and resolved at their root, AI-generated images of any figure from marginalized backgrounds will remain suspect, if not outright dismissed. The rightful demand is for technology to respect truth and accuracy rather than obscure it. Further tolerance of this flawed output signals complicity in a process that whitewashes historical figures and rewrites cultural narratives under the guise of innovation.
© Photo
ChatGPT/Dall-E