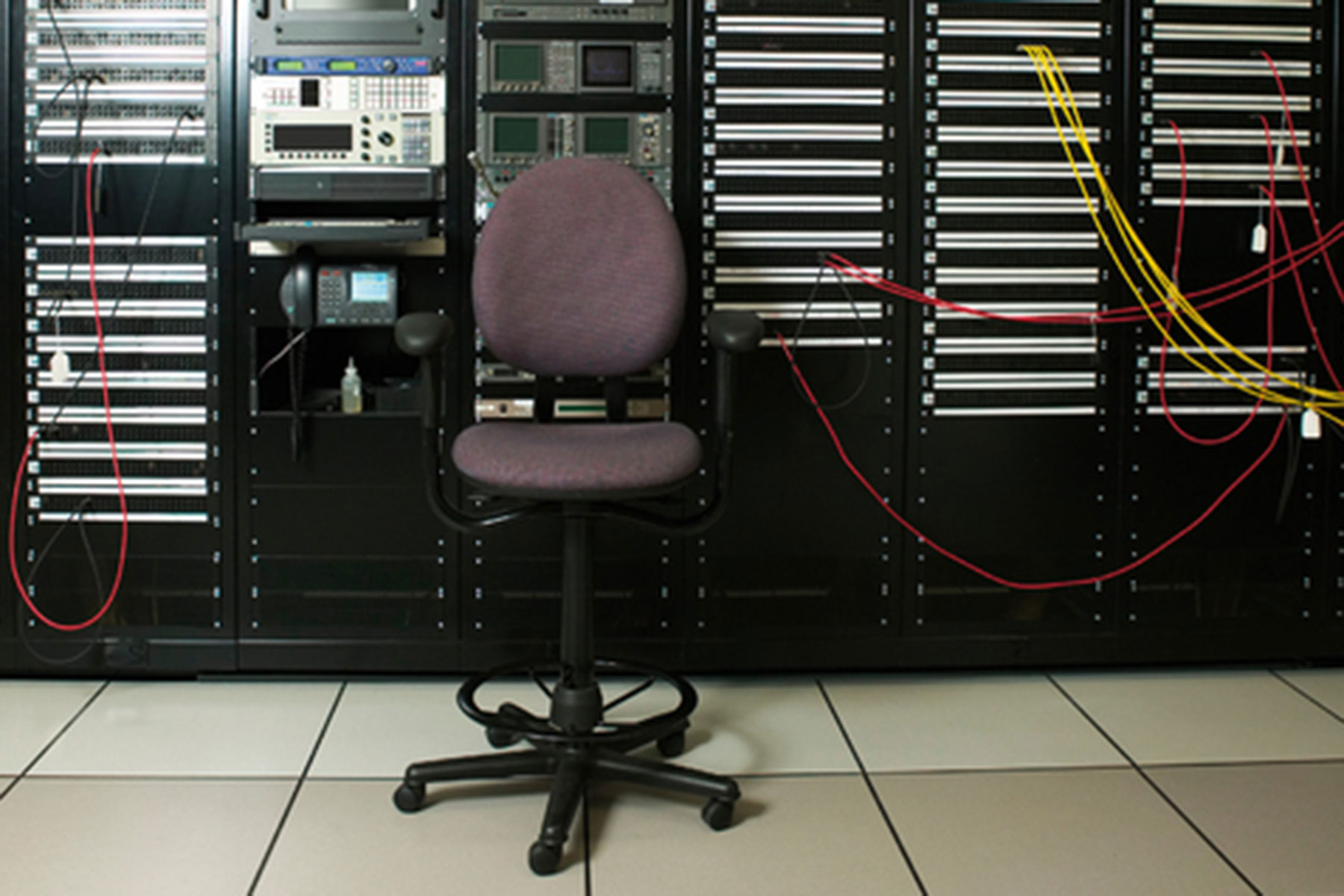
Virginia Eubanks explores the question: are low-income Americans unfairly targeted and punished through digital decision-making tools in her new book, “Automating Inequality: How High-Tech Tools Profile, Police, and Punish the Poor.”
Eubanks will discuss her findings at the Milwaukee Public Library’s Richard E. and Lucille Krug Rare Books Room at 814 W. Wisconsin Avenue, 6:30 p.m. on February 5. Community Advocates Public Policy Institute, the Milwaukee Public Library, and Boswell Book Company. are sponsoring her talk.
In Automating Inequality, Eubanks shines a spotlight on Indiana’s digitized welfare benefits process, screening tools for those experiencing homelessness in Los Angeles, and the information collected on public services seekers in Allegheny County, Pennsylvania.
Of the book, Naomi Klein writes: “This book is downright scary—but with its striking research and moving, indelible portraits of life in the digital poorhouse, you will emerge smarter and more empowered to demand justice.”
Since the dawn of the digital age, decision-making in finance, employment, politics, health and human services has undergone revolutionary change.
Today, automated systems—rather than humans—control which neighborhoods get policed, which families attain needed resources, and who is investigated for fraud. While we all live under this new regime of data, the most invasive and punitive systems are aimed at the poor.
In Automating Inequality, Virginia Eubanks systematically investigates the impacts of data mining, policy algorithms, and predictive risk models on poor and working-class people in America. The book is full of heart-wrenching and eye-opening stories, from a woman whose benefits are literally cut off as she lays dying, to a family in daily fear of losing their daughter because they fit a certain statistical profile.
The U.S. has always used its most cutting-edge science and technology to contain, investigate, discipline and punish the destitute. Like the county poorhouse and scientific charity before them, digital tracking and automated decision-making.
The method hides poverty from the middle-class public and give the nation the ethical distance it needs to make inhumane choices: which families get food and which starve, who has housing and who remains homeless, and which families are broken up by the state. In the process, they weaken democracy and betray our most cherished national values.
Read an Excerpt
INTRODUCTION: Red Flags
In October 2015, a week after I started writing this book, my kind and brilliant partner of 13 years, Jason, got jumped by four guys while walking home from the corner store on our block in Troy, New York. He remembers someone asking him for a cigarette before he was hit the first time. He recalls just flashes after that: waking up on a folding chair in the bodega, the proprietor telling him to hold on, police officers asking questions, a jagged moment of light and sound during the ambulance ride.
It’s probably good that he doesn’t remember. His attackers broke his jaw in half a dozen places, both his eye sockets, and one of his cheekbones before making off with the $35 he had in his wallet. By the time he got out of the hospital, his head looked like a misshapen, rotten pumpkin. We had to wait two weeks for the swelling to go down enough for facial reconstruction surgery. On October 23, a plastic surgeon spent six hours repairing the damage, rebuilding Jason’s skull with titanium plates and tiny bone screws, and wiring his jaw shut.
We marveled that Jason’s eyesight and hearing hadn’t been damaged. He was in a lot of pain but relatively good spirits. He lost only one tooth. Our community rallied around us, delivering an almost constant stream of soup and smoothies to our door. Friends planned a fundraiser to help with insurance co-pays, lost wages, and the other unexpected expenses of trauma and healing. Despite the horror and fear of those first few weeks, we felt lucky.
Then, a few days after his surgery, I went to the drugstore to pick up his painkillers. The pharmacist informed me that the prescription had been canceled. Their system showed that we did not have health insurance.
In a panic, I called our insurance provider. After navigating through their voice-mail system and waiting on hold, I reached a customer service representative. I explained that our prescription coverage had been denied. Friendly and concerned, she said that the computer system didn’t have a “start date” for our coverage. That’s strange, I replied, because the claims for Jason’s trip to the emergency room had been paid. We must have had a start date at that point. What had happened to our coverage since?
She assured me that it was just a mistake, a technical glitch. She did some back-end database magic and reinstated our prescription coverage. I picked up Jason’s pain meds later that day. But the disappearance of our policy weighed heavily on my mind. We had received insurance cards in September. The insurance company paid the emergency room doctors and the radiologist for services rendered on October 8. How could we be missing a start date?
I looked up our claims history on the insurance company’s website, stomach twisting. Our claims before October 16 had been paid. But all the charges for the surgery a week later—more than $62,000—had been denied. I called my insurance company again. I navigated the voice-mail system and waited on hold. This time I was not just panicked; I was angry. The customer service representative kept repeating that “the system said” our insurance had not yet started, so we were not covered. Any claims received while we lacked coverage would be denied.
I developed a sinking feeling as I thought it through. I had started a new job just days before the attack; we switched insurance providers. Jason and I aren’t married; he is insured as my domestic partner. We had the new insurance for a week and then submitted tens of thousands of dollars worth of claims. It was possible that the missing start date was the result of an errant keystroke in a call center. But my instinct was that an algorithm had singled us out for a fraud investigation, and the insurance company had suspended our benefits until their inquiry was complete. My family had been red-flagged.
* * *
Since the dawn of the digital age, decision-making in finance, employment, politics, health, and human services has undergone revolutionary change. Forty years ago, nearly all of the major decisions that shape our lives—whether or not we are offered employment, a mortgage, insurance, credit, or a government service—were made by human beings. They often used actuarial processes that made them think more like computers than people, but human discretion still ruled the day. Today, we have ceded much of that decision-making power to sophisticated machines. Automated eligibility systems, ranking algorithms, and predictive risk models control which neighborhoods get policed, which families attain needed resources, who is short-listed for employment, and who is investigated for fraud.
Health-care fraud is a real problem. According to the FBI, it costs employers, policy holders, and taxpayers nearly $30 billion a year, though the great majority of it is committed by providers, not consumers. I don’t fault insurance companies for using the tools at their disposal to identify fraudulent claims, or even for trying to predict them. But the human impacts of red-flagging, especially when it leads to the loss of crucial life-saving services, can be catastrophic. Being cut off from health insurance at a time when you feel most vulnerable, when someone you love is in debilitating pain, leaves you feeling cornered and desperate.
As I battled the insurance company, I also cared for Jason, whose eyes were swollen shut and whose reconstructed jaw and eye sockets burned with pain. I crushed his pills—painkiller, antibiotic, anti-anxiety medications—and mixed them into his smoothies. I helped him to the bathroom. I found the clothes he was wearing the night of the attack and steeled myself to go through his blood-caked pockets. I comforted him when he awoke with flashbacks. With equal measures of gratitude and exhaustion, I managed the outpouring of support from our friends and family.
I called the customer service number again and again. I asked to speak to supervisors, but call center workers told me that only my employer could speak to their bosses. When I finally reached out to the human resources staff at my job for help, they snapped into action. Within days, our insurance coverage had been “reinstated.” It was an enormous relief, and we were able to keep follow-up medical appointments and schedule therapy without fear of bankruptcy. But the claims that had gone through during the month we mysteriously lacked coverage were still denied. I had to tackle correcting them, laboriously, one by one. Many of the bills went into collections. Each dreadful pink envelope we received meant I had to start the process all over again: call the doctor, the insurance company, the collections agency. Correcting the consequences of a single missing date took a year.
I’ll never know if my family’s battle with the insurance company was the unlucky result of human error. But there is good reason to believe that we were targeted for investigation by an algorithm that detects health-care fraud. We presented some of the most common indicators of medical malfeasance: our claims were incurred shortly after the inception of a new policy; many were filed for services rendered late at night; Jason’s prescriptions included controlled substances, such as the oxycodone that helped him manage his pain; we were in an untraditional relationship that could call his status as my dependent into question.
The insurance company repeatedly told me that the problem was the result of a technical error, a few missing digits in a database. But that’s the thing about being targeted by an algorithm: you get a sense of a pattern in the digital noise, an electronic eye turned toward you, but you can’t put your finger on exactly what’s amiss. There is no requirement that you be notified when you are red-flagged. There is no sunshine law that compels companies to release the inner details of their digital fraud detection systems. With the notable exception of credit reporting, we have remarkably limited access to the equations, algorithms, and models that shape our life chances.
* * *
Our world is crisscrossed with informational sentinels like the system that targeted my family for investigation. Digital security guards collect information about us, make inferences about our behavior, and control access to resources. Some are obvious and visible: closed-circuit cameras bristle on our street corners, our cell phones’ global positioning devices record our movements, police drones fly over political protests. But many of the devices that collect our information and monitor our actions are inscrutable, invisible pieces of code. They are embedded in social media interactions, flow through applications for government services, envelop every product we try or buy. They are so deeply woven into the fabric of social life that, most of the time, we don’t even notice we are being watched and analyzed.
We all inhabit this new regime of digital data, but we don’t all experience it in the same way. What made my family’s experience endurable was the access to information, discretionary time, and self-determination that professional middle-class people often take for granted. I knew enough about algorithmic decision-making to immediately suspect that we had been targeted for a fraud investigation. My flexible work schedule allowed me to spend hours on the phone dealing with our insurance troubles. My employer cared enough about my family’s well-being to go to bat for me. We never stopped assuming we were eligible for medical insurance, so Jason got the care he needed.
We also had significant material resources. Our friends’ fund-raiser netted $15,000. We hired an aide to help Jason return to work and used the remaining funds to defray insurance co-pays, lost income, and increased expenses for things like food and therapy. When that windfall was exhausted, we spent our savings. Then we stopped paying our mortgage. Finally, we took out a new credit card and racked up an additional $5,000 in debt. It will take us some time to recover from the financial and emotional toll of the beating and the ensuing insurance investigation. But in the big picture, we were fortunate.
Not everyone fares so well when targeted by digital decision-making systems. Some families don’t have the material resources and community support we enjoyed. Many don’t know that they are being targeted, or don’t have the energy or expertise to push back when they are. Perhaps most importantly, the kind of digital scrutiny Jason and I underwent is a daily occurrence for many people, not a one-time aberration.
In his famous novel 1984, George Orwell got one thing wrong. Big Brother is not watching you, he’s watching us. Most people are targeted for digital scrutiny as members of social groups, not as individuals. People of color, migrants, unpopular religious groups, sexual minorities, the poor, and other oppressed and exploited populations bear a much higher burden of monitoring and tracking than advantaged groups.
Marginalized groups face higher levels of data collection when they access public benefits, walk through highly policed neighborhoods, enter the health-care system, or cross national borders. That data acts to reinforce their marginality when it is used to target them for suspicion and extra scrutiny. Those groups seen as undeserving are singled out for punitive public policy and more intense surveillance, and the cycle begins again. It is a kind of collective red-flagging, a feedback loop of injustice.
For example, in 2014 Maine Republican governor Paul LePage attacked families in his state receiving meager cash benefits from Temporary Assistance to Needy Families (TANF). These benefits are loaded onto electronic benefits transfer (EBT) cards that leave a digital record of when and where cash is withdrawn. LePage’s administration mined data collected by federal and state agencies to compile a list of 3,650 transactions in which TANF recipients withdrew cash from ATMs in smoke shops, liquor stores, and out-of-state locations. The data was then released to the public via Google Docs.
The transactions that LePage found suspicious represented only 0.03 percent of the 1.1 million cash withdrawals completed during the time period, and the data only showed where cash was withdrawn, not how it was spent. But the governor used the public data disclosure to suggest that TANF families were defrauding taxpayers by buying liquor, lottery tickets, and cigarettes with their benefits. Lawmakers and the professional middle-class public eagerly embraced the misleading tale he spun from a tenuous thread of data.
The Maine legislature introduced a bill that would require TANF families to retain all cash receipts for 12 months to facilitate state audits of their spending. Democratic legislators urged the state’s attorney general to use LePage’s list to investigate and prosecute fraud. The governor introduced a bill to ban TANF recipients from using out-of-state ATMs. The proposed laws were impossible to obey, patently unconstitutional, and unenforceable, but that’s not the point. This is performative politics. The legislation was not intended to work; it was intended to heap stigma on social programs and reinforce the cultural narrative that those who access public assistance are criminal, lazy, spendthrift addicts.
* * *
LePage’s use of EBT data to track and stigmatize poor and working-class people’s decision-making didn’t come as much of a surprise to me. By 2014, I had been thinking and writing about technology and poverty for 20 years. I taught in community technology centers, organized workshops on digital justice for grassroots organizers, led participatory design projects with women in low-income housing, and interviewed hundreds of welfare and child protective services clients and caseworkers about their experiences with government technology.
For the first ten years of this work, I was cautiously optimistic about the impact of new information technologies on economic justice and political vitality in the United States. In my research and organizing, I found that poor and working-class women in my hometown of Troy, New York, were not “technology poor,” as other scholars and policy-makers assumed. Data-based systems were ubiquitous in their lives, especially in the low-wage workplace, the criminal justice system, and the public assistance system. I did find many trends that were troubling, even in the early 2000s: high-tech economic development was increasing economic inequality in my hometown, intensive electronic surveillance was being integrated into public housing and benefit programs, and policy-makers were actively ignoring the needs and insights of poor and working people. Nevertheless, my collaborators articulated hopeful visions that information technology could help them tell their stories, connect with others, and strengthen their embattled communities.
Since the Great Recession, my concern about the impacts of high-tech tools on poor and working-class communities has increased. The skyrocketing economic insecurity of the last decade has been accompanied by an equally rapid rise of sophisticated data-based technologies in public services: predictive algorithms, risk models, and automated eligibility systems. Massive investments in data-driven administration of public programs are rationalized by a call for efficiency, doing more with less, and getting help to those who really need it. But the uptake of these tools is occurring at a time when programs that serve the poor are as unpopular as they have ever been. This is not a coincidence. Technologies of poverty management are not neutral. They are shaped by our nation’s fear of economic insecurity and hatred of the poor; they in turn shape the politics and experience of poverty.
The cheerleaders of the new data regime rarely acknowledge the impacts of digital decision-making on poor and working-class people. This myopia is not shared by those lower on the economic hierarchy, who often see themselves as targets rather than beneficiaries of these systems. For example, one day in early 2000, I sat talking to a young mother on welfare about her experiences with technology. When our conversation turned to EBT cards, Dorothy Allen said, “They’re great. Except [Social Services] uses them as a tracking device.” I must have looked shocked, because she explained that her caseworker routinely looked at her purchase records. Poor women are the test subjects for surveillance technology, Dorothy told me. Then she added, “You should pay attention to what happens to us. You’re next.”
Dorothy’s insight was prescient. The kind of invasive electronic scrutiny she described has become commonplace across the class spectrum today. Digital tracking and decision-making systems have become routine in policing, political forecasting, marketing, credit reporting, criminal sentencing, business management, finance, and the administration of public programs. As these systems developed in sophistication and reach, I started to hear them described as forces for control, manipulation, and punishment. Stories of new technologies facilitating communication and opening opportunity became harder to find. Today, I mostly hear that the new regime of data constricts poor and working-class people’s opportunities, demobilizes their political organizing, limits their movement, and undercuts their human rights. What has happened since 2007 to alter so many people’s hopes and dreams? How has the digital revolution become a nightmare for so many?
* * *
To answer these questions, I set out in 2014 to systematically investigate the impacts of high-tech sorting and monitoring systems on poor and working-class people in America. I chose three stories to explore: an attempt to automate eligibility processes for the state of Indiana’s welfare system; an electronic registry of the unhoused in Los Angeles; and a risk model that promises to predict which children will be future victims of abuse or neglect in Allegheny County, Pennsylvania.
The three stories capture different aspects of the human service system: public assistance programs such as TANF, the Supplemental Nutrition Assistance Program (SNAP), and Medicaid in Indiana; homeless services in Los Angeles; and child welfare in Allegheny County. They also provide geographical diversity: I started in rural Tipton County in America’s heartland, spent a year exploring the Skid Row and South Central neighborhoods of Los Angeles, and ended by talking to families living in the impoverished suburbs that ring Pittsburgh.
I chose these particular stories because they illustrate how swiftly the ethical and technical complexity of automated decision-making has increased in the last decade. The 2006 Indiana eligibility modernization experiment was fairly straightforward: the system accepted online applications for services, checked and verified income and other personal information, and set benefit levels. The electronic registry of the unhoused I studied in Los Angeles, called the coordinated entry system, was piloted seven years later. It deploys computerized algorithms to match unhoused people in its registry to the most appropriate available housing resources. The Allegheny Family Screening Tool, launched in August 2016, uses statistical modeling to provide hotline screeners with a predictive risk score that shapes the decision whether or not to open child abuse and neglect investigations.
I started my reporting in each location by reaching out to organizations working closely with the families most directly impacted by these systems. Over three years, I conducted 105 interviews, sat in on family court, observed a child abuse hotline call center, searched public records, submitted Freedom of Information Act requests, pored through court filings, and attended dozens of community meetings. Though I thought it was important to start from the point of view of poor families, I didn’t stop there. I talked to caseworkers, activists, policy-makers, program administrators, journalists, scholars, and police officers, hoping to understand the new digital infrastructure of poverty relief from both sides of the desk.
What I found was stunning. Across the country, poor and working-class people are targeted by new tools of digital poverty management and face life-threatening consequences as a result. Automated eligibility systems discourage them from claiming public resources that they need to survive and thrive. Complex integrated databases collect their most personal information, with few safeguards for privacy or data security, while offering almost nothing in return. Predictive models and algorithms tag them as risky investments and problematic parents. Vast complexes of social service, law enforcement, and neighborhood surveillance make their every move visible and offer up their behavior for government, commercial, and public scrutiny.
These systems are being integrated into human and social services across the country at a breathtaking pace, with little or no political discussion about their impacts. Automated eligibility is now standard practice in almost every state’s public assistance office. Coordinated entry is the preferred system for managing homeless services, championed by the United States Interagency Council on Homelessness and the U.S. Department of Housing and Urban Development. Even before the Allegheny Family Screening Tool was launched, its designers were in negotiations to create another child maltreatment predictive risk model in California.
Though these new systems have the most destructive and deadly effects in low-income communities of color, they impact poor and working-class people across the color line. While welfare recipients, the unhoused, and poor families face the heaviest burdens of high-tech scrutiny, they aren’t the only ones affected by the growth of automated decision-making. The widespread use of these systems impacts the quality of democracy for us all.
Automated decision-making shatters the social safety net, criminalizes the poor, intensifies discrimination, and compromises our deepest national values. It reframes shared social decisions about who we are and who we want to be as systems engineering problems. And while the most sweeping digital decision-making tools are tested in what could be called “low rights environments” where there are few expectations of political accountability and transparency, systems first designed for the poor will eventually be used on everyone.
America’s poor and working-class people have long been subject to invasive surveillance, midnight raids, and punitive public policy that increase the stigma and hardship of poverty. During the nineteenth century, they were quarantined in county poorhouses. During the twentieth century, they were investigated by caseworkers, treated like criminals on trial. Today, we have forged what I call a digital poorhouse from databases, algorithms, and risk models. It promises to eclipse the reach and repercussions of everything that came before.
Like earlier technological innovations in poverty management, digital tracking and automated decision-making hide poverty from the professional middle-class public and give the nation the ethical distance it needs to make inhuman choices: who gets food and who starves, who has housing and who remains homeless, and which families are broken up by the state. The digital poorhouse is part of a long American tradition. We manage the individual poor in order to escape our shared responsibility for eradicating poverty.
Copyright © 2017 by Virginia Eubanks
© Photo
Sadaf Rassoul Cameron